AI Sustainability: Reducing Carbon Footprint and Driving Innovation
As AI technology expands, so does its carbon footprint. This underscores the challenge: while AI accelerates digital transformation, it risks becoming a significant contributor to global CO₂ emissions.
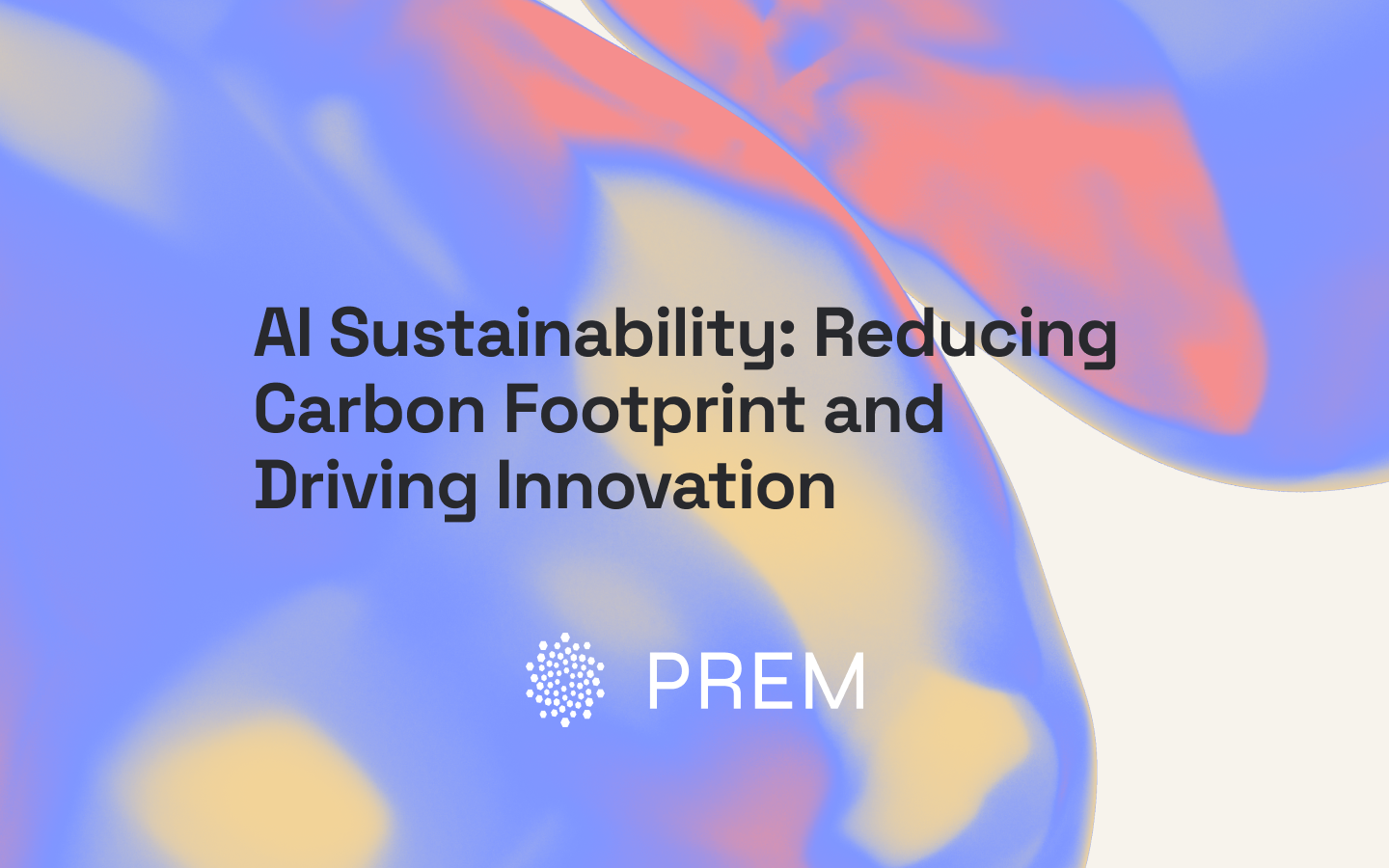
The Hidden Environmental Cost of AI
Artificial Intelligence (AI) has emerged as a transformative force, driving innovation across industries and enhancing productivity. However, as AI adoption grows, so does a critical and often overlooked consequence: its environmental impact. The computational power required to train large-scale AI models, such as those used in machine learning (ML), results in significant energy consumption and carbon emissions. While AI holds the potential to address global challenges, its environmental costs, if left unchecked, could ultimately counteract these benefits.
A particular concern is the rapidly increasing carbon footprint of data centers, which house the vast infrastructure needed for AI operations. These centers consume enormous amounts of electricity, often matching the energy demands of entire cities. In fact, some data centers now surpass the airline industry in terms of carbon emissions, highlighting the urgent need to address AI's environmental toll.
As AI technology expands, so does its carbon footprint. For example, training a single large AI model can emit over 626,000 pounds of CO₂—nearly five times the lifetime emissions of an average car. This underscores the challenge: while AI accelerates digital transformation, it risks becoming a significant contributor to global CO₂ emissions.
The combination of massive energy demands for training and deploying AI models, alongside the reliance on data centers powered by non-renewable energy, makes the environmental cost of AI an urgent issue. To balance AI's benefits with its environmental footprint, the industry must adopt sustainable practices that align AI development with global climate goals.
Training AI Models: The Carbon Footprint of Machine Learning
Training large-scale AI models is a highly energy-intensive process. Models like GPT-3 and other advanced machine learning systems require vast computational resources to process massive datasets during training, resulting in significant energy consumption. This high demand is compounded by the necessity of running complex neural networks through multiple iterations to achieve optimal performance. As a result, training a single large model can emit more than 626,000 pounds of CO₂, equivalent to the lifetime emissions of several cars.
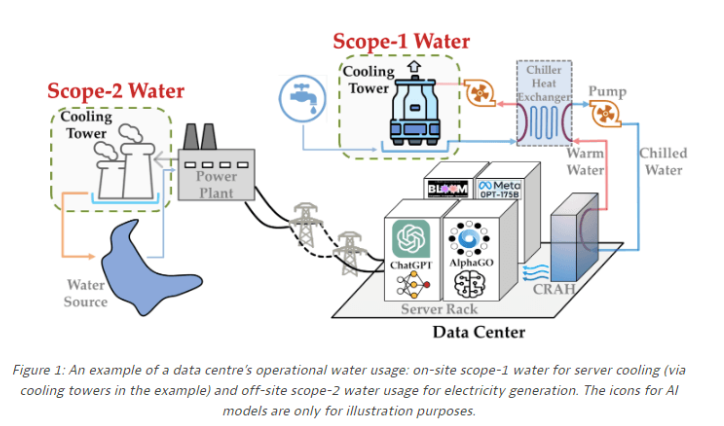
Data centers that support AI workloads contribute heavily to greenhouse gas emissions. The cooling systems necessary to maintain these vast infrastructures consume significant water resources, as illustrated above. AI-related infrastructure could soon consume six times more water than the entire country of Denmark. This is a significant concern, especially in regions where water scarcity is already a pressing issue. Water is not only used for cooling during data center operations but also during the construction phase of these facilities, exacerbating the environmental toll.
In addition to water consumption, the production of AI-related electronic waste (e-waste) poses another challenge. Data centers produce large amounts of e-waste, including hazardous substances like mercury and lead, which can have long-term detrimental effects on the environment if not properly managed. The rapid growth in the number of AI-driven data centers, which has surged from 500,000 to 8 million globally since 2012, has dramatically increased the scale of e-waste production.
These emissions stem not just from the raw computational energy but also from the cooling systems required to maintain optimal server performance in data centers, which generate massive amounts of heat during operations.
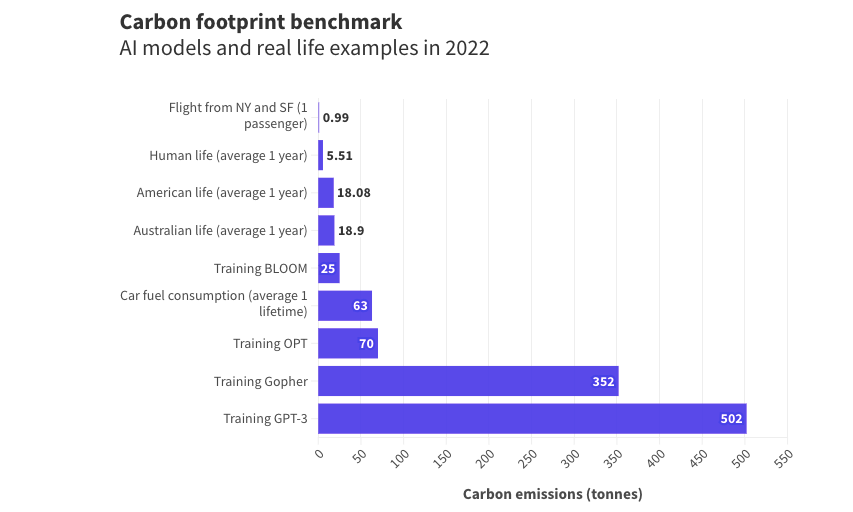
As the chart above shows, training a model like GPT-3 can result in emissions as high as 502 tonnes of CO2, significantly higher than the lifetime emissions of an average car, which is around 63 tonnes. This stark comparison highlights the environmental cost of large AI models compared to everyday activities, such as a transatlantic flight or a single human’s annual carbon footprint.
The challenges posed by the energy demands of AI training are compounded by the growing trend toward developing increasingly large models, a phenomenon sometimes described as the "bigger is better" approach. This strategy, while leading to cutting-edge advancements in AI capabilities, also results in diminishing returns when it comes to environmental efficiency. As models become larger, the additional energy needed for their training does not correspond to proportional improvements in their performance(Sustainable AI).
Thus, while AI's promise remains unparalleled, the current trajectory of AI model development is unsustainable from an environmental perspective. If the industry continues to prioritize scale without considering energy efficiency, the carbon cost of AI could counteract global efforts to combat climate change.
Sustainable AI Solutions: Minimizing Environmental Impact
As AI continues to evolve, mitigating its environmental impact is paramount. The vast energy consumption associated with training AI models can be reduced through several strategies that focus on more efficient AI development and deployment. From optimizing AI architecture to using greener energy sources, these solutions are critical for ensuring that AI's growth does not exacerbate the global climate crisis.
Model Compression and Efficient Architectures
Optimizing hardware usage is one of the primary ways to reduce the environmental toll of AI. Techniques such as model compression and quantization significantly lower the energy consumption of AI systems. By reducing the size of AI models, developers can decrease the computational resources needed to process them, thus cutting down the overall energy expenditure. This approach not only improves model efficiency but also allows AI to be deployed on resource-constrained devices.
Sustainable Data Centers and Cloud Computing
Data centers, essential for running AI workloads, consume vast amounts of energy, much of it derived from non-renewable sources. To address this, companies are increasingly moving their operations to more energy-efficient data centers and exploring renewable energy options like wind, solar, or hydroelectric power. For instance, data centers in regions like Montreal, Canada, benefit from access to hydroelectricity as a primary energy source, making it a potential model for green energy integration in AI operations.
However, another critical aspect of sustainability is managing the resources required to build AI infrastructure. AI models rely on specialized microchips and electronic components that are often made from rare earth minerals, which are frequently mined in environmentally destructive ways. The growing demand for these materials raises concerns about the long-term sustainability of AI hardware production. Implementing more efficient mining practices and focusing on recycling existing materials could help reduce the environmental burden of AI’s resource consumption.
Fine-Tuning and Specialized Hardware
Fine-tuning pre-existing models instead of training models from scratch is another way to reduce the environmental impact of AI. Fine-tuning requires far less computational power and can effectively adapt foundation models to specific tasks without the need for excessive energy consumption. Additionally, the use of specialized hardware such as in-memory computing and analog computing can enhance energy efficiency.
A New Paradigm for AI Development
As AI becomes more integrated into industries worldwide, there is a growing emphasis on finding sustainable energy solutions to power this growth. The adoption of nuclear energy, alongside the optimization of AI architectures and data center operations, represents a promising path toward reducing AI's carbon footprint. These advancements are vital to ensuring that AI's progress is environmentally responsible, balancing innovation with sustainability goals.
By adopting these practices, the AI industry can move toward a more sustainable future, where innovation is balanced with environmental responsibility. However, achieving widespread implementation of these solutions will require a collective effort from developers, organizations, and policymakers to prioritize sustainability at every stage of the AI lifecycle.
AI as a Tool for Environmental Sustainability
While AI’s environmental footprint poses challenges, it also holds the potential to be a powerful tool for driving environmental sustainability. AI technologies are already being leveraged to optimize renewable energy systems, enhance waste management, and combat climate change. Through advanced data analytics and machine learning algorithms, AI can contribute to building a more sustainable future by transforming how we monitor, manage, and conserve natural resources.
Optimizing Renewable Energy Systems
AI plays a critical role in improving the efficiency and productivity of renewable energy sources such as wind and solar power. By analyzing weather patterns, energy loads, and system performance, AI-driven models can help optimize the generation and distribution of renewable energy. This ensures that energy resources are used more efficiently, reducing waste and maximizing output. For example, AI has been used to enhance the eco-friendliness of solar and wind farms, ensuring that renewable energy systems operate at peak performance.
Managing Waste and Resources
AI can also support the implementation of circular economy principles by improving resource efficiency and waste management. Machine learning models can track waste production and identify patterns, allowing for better waste segregation, recycling, and reuse. This helps minimize environmental impact by reducing the amount of waste that ends up in landfills and promoting more sustainable consumption and production practices. AI’s ability to analyze large datasets can inform more effective waste management policies and foster environmentally friendly practices across industries.
Addressing Climate Change
Perhaps one of AI’s most promising contributions to sustainability is its potential to aid in climate change mitigation. AI-based models can process extensive climate data to uncover patterns and correlations that inform policy decisions and adaptation strategies. For instance, machine learning algorithms can develop simulations to forecast the impacts of climate change, allowing governments and organizations to better prepare for and respond to environmental challenges. These models are instrumental in creating comprehensive climate action plans that address everything from carbon emissions to biodiversity loss.
AI also plays a role in ecological conservation, with AI-powered systems being used to monitor wildlife, forests, and oceans. By analyzing data from satellites, drones, and sensors, AI can detect illegal deforestation, track endangered species, and even predict natural disasters. This contributes to global conservation efforts aimed at preserving biodiversity and protecting ecosystems.
In summary, while AI development needs to address its own environmental costs, it simultaneously offers significant opportunities for advancing sustainability. Through its ability to optimize renewable energy, manage resources, and address climate change, AI is proving to be an essential tool in the fight for a more sustainable planet.
Ethical AI Development: Transparency and Accountability
In addition to transparency, sustainability must be a cornerstone of ethical AI development. AI systems, particularly those that require large-scale data processing and energy-intensive operations, contribute significantly to global carbon emissions. To mitigate these environmental impacts, AI developers must adopt sustainable practices throughout the AI lifecycle. This includes using energy-efficient hardware, optimizing model architectures, and transferring workloads to energy-efficient data centers.
Governments and tech companies alike must also take responsibility for managing AI’s electronic waste and resource consumption. AI infrastructure generates massive amounts of e-waste, including toxic substances like mercury and lead, which require careful management to avoid environmental harm. Policymakers can help by setting standardized procedures for measuring the environmental impact of AI, encouraging transparency from companies about the ecological cost of their AI models and services.
As AI continues to evolve and expand its influence across industries, ensuring ethical and transparent development practices is essential. AI systems have the potential to drive innovation and enhance sustainability, but without proper governance and accountability frameworks, these advancements may come at a high social and ethical cost. Transparency in AI development is critical not only for understanding how AI models make decisions but also for ensuring that these decisions align with ethical standards and do not exacerbate inequalities or environmental harm.
The Need for Transparency in AI Models
The complexity of AI models, particularly large generative models, often obscures the decision-making processes that underpin their outputs. This lack of transparency can lead to unintended biases, unfair outcomes, and even legal challenges, as seen in recent cases of copyright infringement involving AI systems. To address these issues, frameworks for AI governance must include transparency as a core principle, ensuring that AI models are understandable and accountable.
Efforts like the development of the Foundation Model Transparency Index, designed by researchers from Stanford, MIT, and Princeton, aim to create standardized methods for evaluating AI model transparency. This scoring system assesses how AI models are built, how they function, and how they are used in various applications. Such tools are essential for fostering trust in AI systems and ensuring that they operate in ways that are fair, equitable, and aligned with societal values.
Sustainability in AI Development
In addition to transparency, sustainability must be a cornerstone of ethical AI development. AI systems, particularly those that require large-scale data processing and energy-intensive operations, contribute significantly to global carbon emissions. To mitigate these environmental impacts, AI developers must adopt sustainable practices throughout the AI lifecycle. This includes using energy-efficient hardware, optimizing model architectures, and transferring workloads to energy-efficient data centers.
Governance Frameworks and Ethical Standards
To ensure that AI systems are developed and deployed responsibly, robust governance frameworks must be established. These frameworks should address critical issues such as accountability, data privacy, and fairness. The Montreal Declaration for Responsible AI and the Organisation for Economic Co-operation and Development’s AI Principles offer widely accepted guidelines for promoting ethical AI development.
Furthermore, public-private partnerships and interdisciplinary collaboration are crucial for creating comprehensive solutions that integrate ethical considerations into AI governance. By involving diverse stakeholders from academia, industry, and government, AI policies can be shaped to ensure that the technology is used responsibly and for the benefit of society at large.
In summary, transparency and accountability are foundational to ethical AI development. By prioritizing these values alongside sustainability, the AI industry can ensure that its innovations align with societal goals and contribute positively to global progress. Without these safeguards, AI risks perpetuating harm rather than solving the challenges it seeks to address.
Charting the Course Towards Sustainable AI
As the role of artificial intelligence continues to expand, the need for sustainable development practices becomes increasingly urgent. AI, while offering transformative potential in industries ranging from healthcare to environmental management, must evolve to address its own environmental and ethical challenges. The adoption of sustainable AI practices, including energy-efficient model design, transparent governance frameworks, and the use of renewable resources, is crucial for minimizing the negative impacts of AI technologies on our planet.
The growing reliance on large-scale AI models and data centers has significantly increased the energy consumption and carbon footprint of the AI sector. As highlighted, data centers supporting AI workloads now consume as much electricity as entire countries, and the exponential growth of data and AI applications threatens to exacerbate the global climate crisis. To counter this, industry leaders, policymakers, and researchers must collaborate to implement bold initiatives that prioritize sustainability in AI development.
In particular, transitioning to energy-efficient data centers and adopting more sustainable hardware can drastically reduce the carbon footprint of AI operations. Furthermore, the development of smaller, more efficient models—drawing inspiration from the human brain’s energy efficiency—can provide a path toward reducing AI’s energy consumption. These advancements in model design and architecture are vital to ensuring that AI’s progress does not come at the cost of environmental degradation.
Moreover, the ethical dimensions of AI, including transparency, accountability, and fairness, must remain at the forefront of AI governance. By adhering to principles such as those outlined in the Montreal Declaration for Responsible AI, the industry can safeguard against unintended societal harms while promoting trust and accountability in AI systems.
References
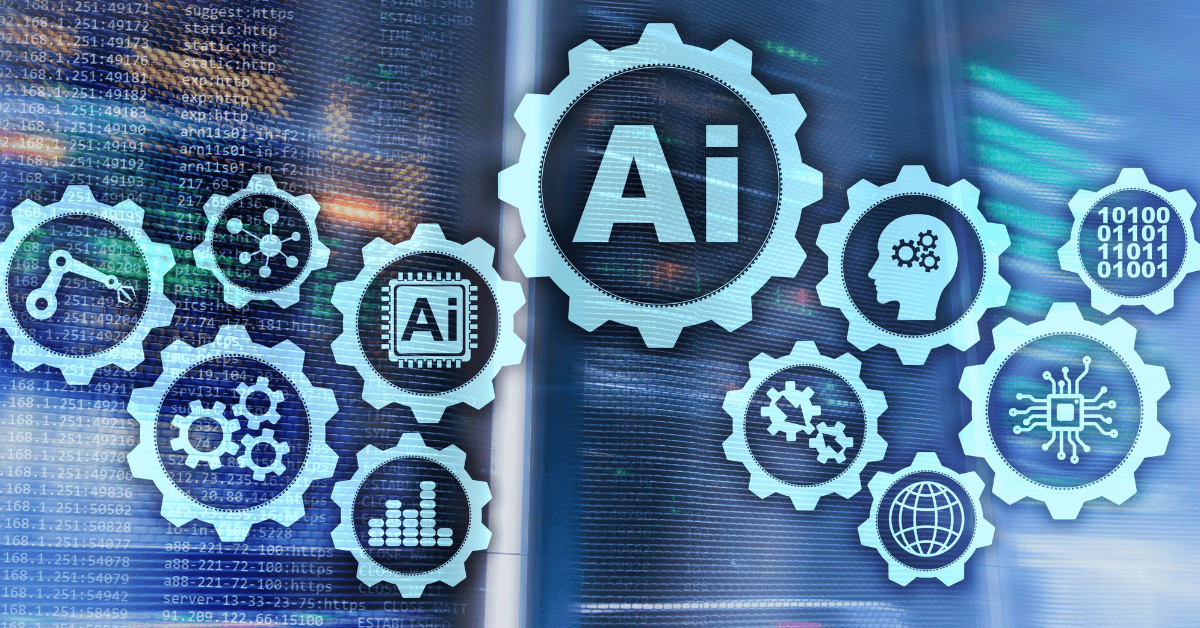

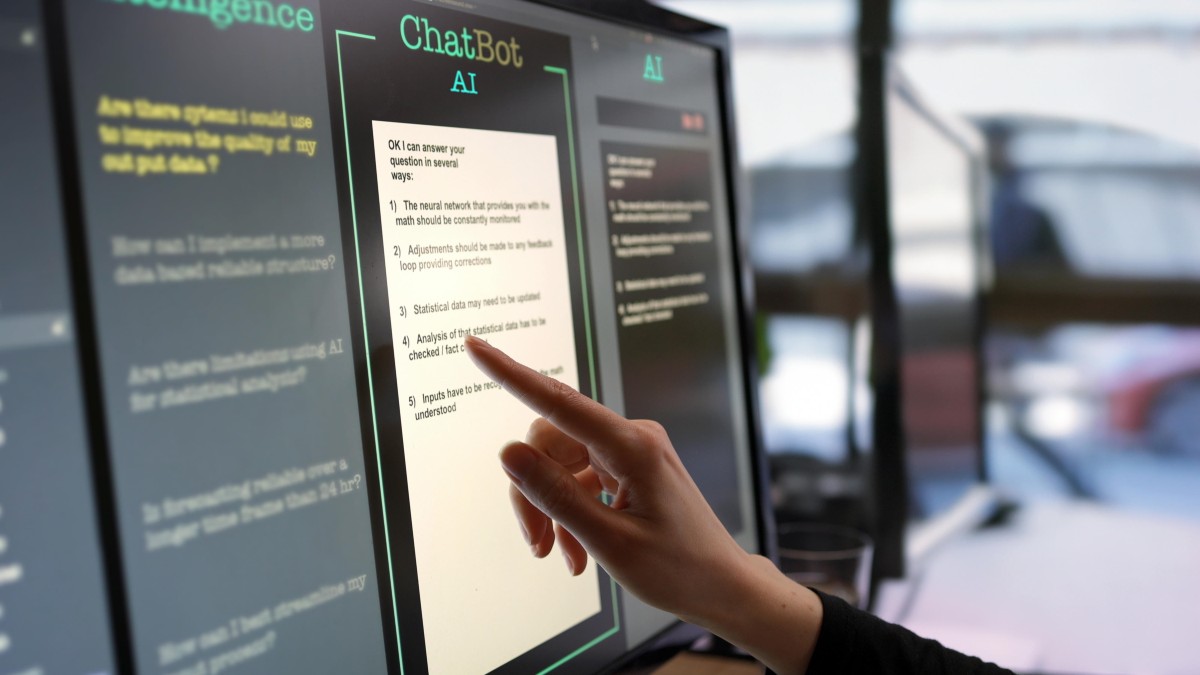